Skyler H. Hoang 1*; Emerson My Lam 2; Hue Dao 1
1, Department of Cell Biology and Neuroscience, Rutgers University, Piscataway, NJ 08854, United States
2, Faculty of Pharmacy, Ton Duc Thang University, Ho Chi Minh City, Viet Nam
E-mail:
hung.hoang@rutgers.edu
Received: 03/10/2023
Acceptance: 19/10/2023
Available Online: 19/10/2023
Published: 01/04/2024
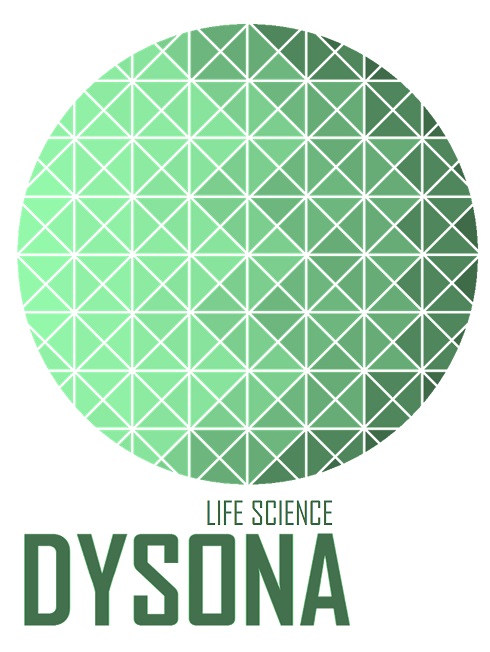
Manuscript link
http://dx.doi.org/10.30493/DLS.2023.419136
Abstract
Emerging evidence suggests that gut microbiota metabolites can have a potential role in conferring neuroprotective benefits against Alzheimer’s disease. Enterolactone, (S)-equol, and urolithin A are bacterial metabolites that originate from rich dietary sources. In this study, the inhibition potential of the aforementioned metabolites on Aβ42 peptide was investigated through molecular dynamics simulations. The study revealed that interacting with metabolites decreases the intra-connectivity of Aβ42, resulting in a reduction in strongly correlated residue pairs and a complete absence of strongly anti-correlated residue pairs. The MM/PBSA binding energy analyses showed that enterolactone had the strongest binding affinity with Aβ42 peptide (-291.0 kJ/mol), while (S)-equol and urolithin A had weaker binding affinities. The study also revealed that enterolactone and (S)-equol have the potential to induce structural perturbations in the Aβ42 peptide, resulting in reduced conformational fluctuations and flexibility. The study suggests that diets rich in matairesinol may inhibit Aβ42 action, as enterolactone is a matairesinol-derived metabolite generated through gut microbiota activity. Additional research is required in order to have a comprehensive understanding of the specific processes that underlie these associations and to develop efficacious supplementary therapy strategies that involve the modulation of gut microbiota and their metabolites.
Keywords: Alzheimer’s disease, Aβ42, Enterolactone, Molecular docking, Molecular dynamics simulation
Introduction
Alzheimer’s disease (AD) is a neurodegenerative ailment that impacts a global population of about 44 million individuals [1]. The disease is typified by the accumulation of amyloid beta peptide 42 (Aβ42) fibrils, leading to cognitive decline and memory loss [2]. Notwithstanding the increasing incidence of AD, the discovery of efficacious therapies remains a daunting challenge, necessitating the exploration of alternative strategies for the prevention and treatment of this ailment. In contemporary scientific research, there has been a significant emphasis placed on investigating the prospective function of phytochemicals, namely matairesinol, daidzein, and ellagic acid [3][4]. These compounds are plentifully present in diets that are enriched with plant-based foods, fruits, and nuts, which are deemed to be nutritionally rich and are associated with a plethora of health benefits, including reduced susceptibility to chronic diseases, such as cardiovascular diseases and certain cancers, enhanced metabolic function, and improved overall well-being [5-10]. These diets also have been identified as critical contributors towards conferring neuroprotective benefits against AD or ameliorating the manifestations of this disease [11-13].
The neuroprotective characteristics of phytochemicals in food are thought to be mediated by many mechanisms such as antioxidant, anti-inflammatory, and anti-amyloidogenic activities. Phytochemicals have been identified as antioxidant molecules capable of counteracting the harmful effects of free radicals and reactive oxygen species, which have the potential to induce cellular damage, including neurological impairment [14][15]. Phytochemicals can modulate the production of pro-inflammatory cytokines, enzymes, and other molecules that contribute to inflammation [16][17]. Finally, the anti-amyloidogenic properties of some phytochemicals involve the modulation of the formation and accumulation of beta-amyloid plaques, which are the hallmark features of AD pathology [18][19].
Recent research indicates that the gut microbiota plays a significant role in the metabolic processes associated with phytochemicals. Particularly, matairesinol, daidzein, and ellagic acid are transformed by gut bacteria into smaller, more bioavailable compounds, such as enterolactone, S-equol, and urolithin A [20-23]. Enterolactone (Fig. 1 A) (a phytoestrogen produced from the bacterial conversion of lignans found in flaxseed, whole grains, and certain fruits and vegetables) may have a protective effect against breast and other hormone-dependent cancers due to its ability to bind to estrogen receptors and modulate hormone activity [24][25]. (S)-equol (Fig. 1 B) (a metabolite produced from the bacterial conversion of daidzein, which is found in soybeans and other legumes) has been shown to have potential benefits for menopausal women, including the relief of hot flashes and improvement in bone health [22][26]. Urolithin A (Fig. 1 C) (a metabolite produced from the bacterial conversion of ellagitannins present in various fruits, including pomegranates, strawberries, and raspberries) has potential benefits for skeletal muscle health, including the promotion of mitochondrial biogenesis and the inhibition of muscle wasting [27][28].
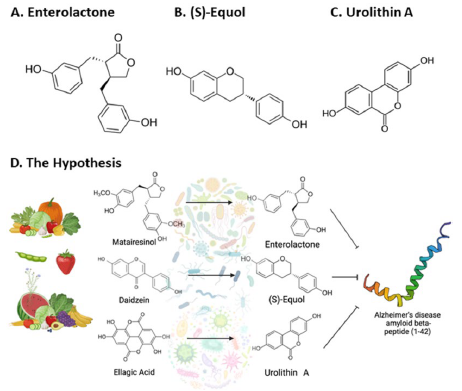
These metabolites have also demonstrated neuroprotective effects, further supporting the potential benefits of consuming a diet rich in these phytochemicals [29-31]. One possible mechanism by which phytochemical compounds like these may confer their neuroprotective effects is through direct interaction with Aβ42, minimizing fibril aggregation and mitigating the pathological features of AD [32][33]. Moreover, emerging research indicates that an imbalance in gut microbiota, known as gut microbiota dysbiosis, has a role in the onset and progression of AD through the acceleration of neuroinflammation, facilitation of senile plaque formation, and alteration of neurotransmitter synthesis [34-36]. Understanding the complex interplay between dietary phytochemicals, gut microbiota, and AD pathophysiology may provide novel insights into the development of therapeutic strategies to prevent or slow the progression of this devastating disease. In this study, it was hypothesized that parent phytochemicals found in plant-based diets of high quality such as matairesinol, daidzein, and ellagic acid exert neuroprotective effects by being transformed into smaller metabolites (enterolactone, (S)-equol, and urolithin A) with higher bioavailability, which would ultimately interact with Aβ42 peptides to minimize plaque formation (Fig. 1 D). Therefore, molecular dynamics simulations were utilized to investigate the inhibition mechanisms of enterolactone, (S)-equol, and urolithin A on the Aβ42 peptides.
Materials and Methods
Ligand retrieval and molecular docking
The 3D structures of Enterolactone, (S)-equol, and urolithin A format were obtained in structure data file (.sdf) format from PubChem using the following CIDs: 114739, 91469, and 5488186, respectively. YASARA Structure [37] was employed to execute molecular docking to the Aβ42 peptide which was retrieved from the Research Collaboratory for Structural Bioinformatics (RCSB) with protein data bank (PDB) identification of 1IYT. The “Clean” function of YASARA Structure was utilized for atom and residue modification, error correction, and structural refinement. Additionally, it was utilized to prepare the PDB file of the Aβ42 peptide for molecular docking, thereby improving the accuracy and quality of the docking process. The dock_run.mcr script was utilized to perform molecular docking of the metabolites against the prepared Aβ42 peptide. This pre-made script serves as an input file for executing molecular docking using the Vina algorithm with pre-defined optimized parameters. One hundred docking runs were conducted for each metabolite with the peptide, and the docked pose exhibiting the highest negative binding affinity (ΔG) in kcal/mol was chosen for subsequent analysis in molecular dynamics simulations.
Molecular dynamics simulation
Molecular dynamics simulations were conducted utilizing established protocols in YASARA Structure [38][39]. It is noteworthy that each simulation was sustained for a duration of 200 nanoseconds and produced 501 snapshots. The simulations employed the AMBER14 force field and were executed at a temperature of 298 Kelvin with a physiological concentration of 0.9% NaCl.
The Dynamic Cross-Correlation Matrix (DCCM) is a fundamental tool used to examine the correlation between the movements of selected pairs of atoms or residues in a protein system. This matrix contains values that range from -1, which indicates perfect anti-correlation, to +1, which represents perfect correlation. In the present study, the DCCM was used to investigate the correlation between residue pairs. This analysis is readily available within YASARA Structure and can be employed immediately following molecular dynamics simulations. A residue pair that displays a value less than -0.8 is considered strongly anti-correlated, indicating that the movements of the two residues are strongly inversely related. Conversely, a residue pair with a value greater than 0.8 is considered strongly correlated, signifying that the movements of the two residues are highly correlated. It is noteworthy that the values along the diagonal of the DCCM are always +1, as the motion of an atom is perfectly correlated with itself. The DCCM analysis provides valuable information on the collective motions of protein residues, which can aid in the identification of key regions involved in protein function and dynamics.
Free energy landscapes
The process of constructing a free energy landscape has been previously documented in the literature [38][40]. The approach in this study involves the application of the Boltzmann-inverting multi-dimensional histogram onto two input variables (namely root-mean-square deviation of the C-alpha atoms (RMSD-Calpha) and radius of gyration), using the ‘gmx sham’ function within GROMACS 2023.2. RMSD-Calpha measures the distance between the positions of the C-alpha atoms of a protein in each conformation compared to a reference structure. It is commonly used to assess the structural similarity of protein structures. The radius of gyration, on the other hand, measures the compactness of the protein by calculating the distance of each atom from the center of mass of the protein. By utilizing these two input variables, a free energy landscape can be constructed, which can provide insights into the conformational space that a protein can access and the relative stability of different protein conformations. This method has proven to be a valuable tool in the study of protein folding, misfolding, and aggregation, as well as in the design of novel therapeutics targeting protein-protein interactions. BIOVIA Discovery Studio 2021 Client was used to analyze the residual interaction of the ligands and the peptide in 2D.
Binding energy derived from MM/PBSA
The md_analysebindenergy.mcr script was utilized to extract the molecular mechanics-Poisson-Boltzmann-derived binding energy (MM/PBSA) based on the following fundamental premise:
Binding Energy = Complex Solvation Energy + Complex Potential Energy – Ligand Solvation Energy – Ligand Potential Energy – Receptor Solvation Energy – Receptor Solvation Energy (kJ/mol)
In this equation, a more negative binding energy implies a stronger binding affinity between the ligand and the receptor, while a more positive binding energy does not necessarily indicate the absence of binding. The MM/PBSA method is widely employed in the study of protein-ligand interactions and is a valuable tool in drug design and discovery. It provides an estimation of the free energy change upon the formation of a protein-ligand complex, which can aid in the identification of potential drug candidates and the optimization of lead compounds. The MM/PBSA approach considers the contributions of various energy components, including electrostatic interactions, van der Waals forces, and solvation effects, which can provide a comprehensive view of the binding mechanism.
Results
Molecular dynamics simulations of Aβ42 complexes
After 80 nanoseconds from the initiation of the molecular dynamics simulations, it was seen that the apo-Aβ42 protein displayed disorder in both its C- and N-termini. Similarly, the Aβ42-urolithin A complex displayed a similar disorder after 80 nanoseconds of simulation initiation. In contrast, both Aβ42-enterolactone and Aβ42-(S)-equol complexes induced a reduction in this disorder by linearly elongating the peptide (Fig. 2).
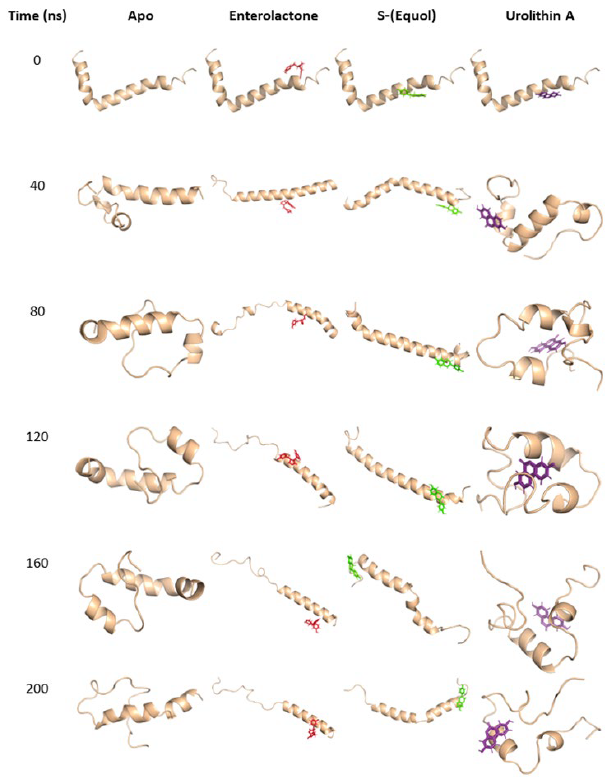
Figure 2. Temporal progression of molecular dynamics simulations. Depiction of apo-Aβ42 and its complexes with the phytochemical metabolites enterolactone, (S)-equol, and urolithin A. The visual representations capture the dynamic changes in the interactions between Aβ42 and the investigated metabolites over the course of the simulation (200 nanoseconds), providing insights into their potential inhibitory effects on Aβ42 aggregation.
Secondary structure analyses of Aβ42 complexes
The graphical representations illustrate the protein secondary structure per residue as a function of simulation time, offering a quantitative insight into the alterations of Aβ42 in all forms throughout the molecular dynamics simulations (Fig. 3). Over a duration of 200 ns, the apo-Aβ42 simulation exhibited 53.26% helix, 0.024% sheet, 7.927% turn, 36.056% coil, and 2.733% helix310 structures (Fig. 3 A). In the Aβ42-enterolactone complex, the distribution was 59.305% helix, 0.019% sheet, 13.706% turn, 25.587% coil, and 1.383% helix310 structures (Fig. 3 B). For the Aβ42-(S)-equol complex, the percentages were 69.257% helix, 0.01% sheet, 10.313% turn, 18.658% coil, and 1.763% helix310 structures (Fig. 3 C). Lastly, in the Aβ42-urolithin A complex, the structure composition was 24.522% helix, 0.171% sheet, 28.134% turn, 42.325% coil, and 4.847% helix310 structures (Fig. 3 D). Helix-pi structures were absent in both the apo-protein and all complexes. It was also noticed that both enterolactone and (S)-equol induced a decrease in coil structure and an increase in turn structure compared to the apo-form. On the other hand, urolithin A exhibited an increase in all secondary structure components at the expense of the helix structure, which underwent a substantial decrease in the Aβ42-urolithin A complex.
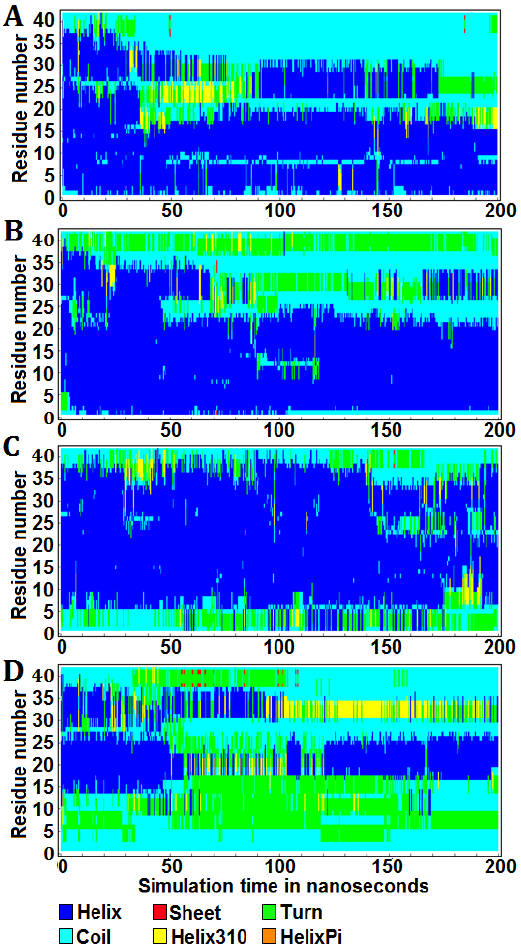
DCCM analyses of Aβ42 complexes
The DCCM analyses revealed that interacting with the metabolites decreased the intra-connectivity of Aβ42 (Fig. 4). Overall, the apo-Aβ42 had 89 strongly correlated residue pairs and 17 strongly anticorrelated residue pairs (Fig. 4 A). In contrast, Aβ42 in complex with enterolactone, (S)-equol, and urolithin A had 9, 14, and 33 strongly correlated residue pairs, respectively, with no strongly anti-correlated residue pairs (Fig. 4 B, C, and D). This suggests that the
interaction of Aβ42 with the metabolites led to a reduction in the number of strongly correlated residue pairs and a complete absence of strongly anti-correlated residue pairs.
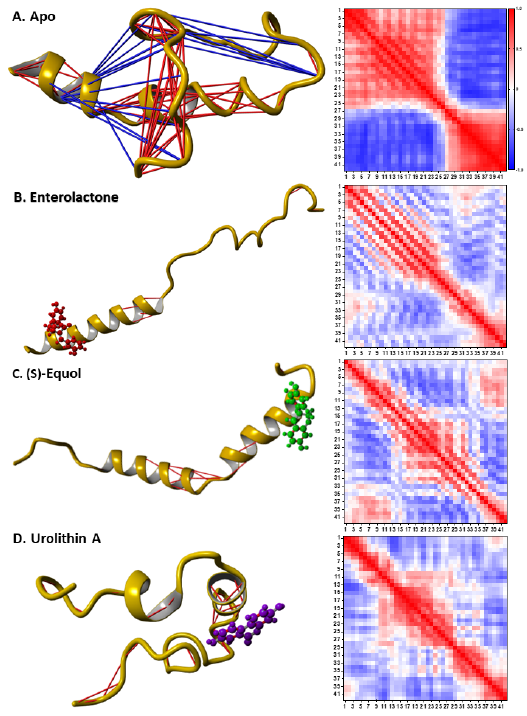
Free energy landscapes of Aβ42 complexes
Free energy landscapes were constructed from two variables, RMSD-Calpha and radius of gyration (Fig. 5). The apo-form reached a meta-stable state at 56.8 ns, with a predicted energy cost of 10.6 kJ/mol to reach the most unstable state from the meta-stable state (Fig. 5 A). The Aβ42-enterolactone and -(S)-equol complexes (which both required 7.87 kJ/mol to reach the most unstable state from meta-stable states) reached meta-stable states at 137.2 ns and 22.0 ns, respectively (Fig. 5 B and C). Interestingly, the Aβ42-urolithin A complex required the highest amount of energy, which was 11.6 kJ/mol (Fig. 5 D). These results indicate that the Aβ42-urolithin A complex has the fewest conformations and can be considered generally stable, while the Aβ42-enterolactone and -(S)-equol complexes have the largest number of conformations and are considered generally more unstable.
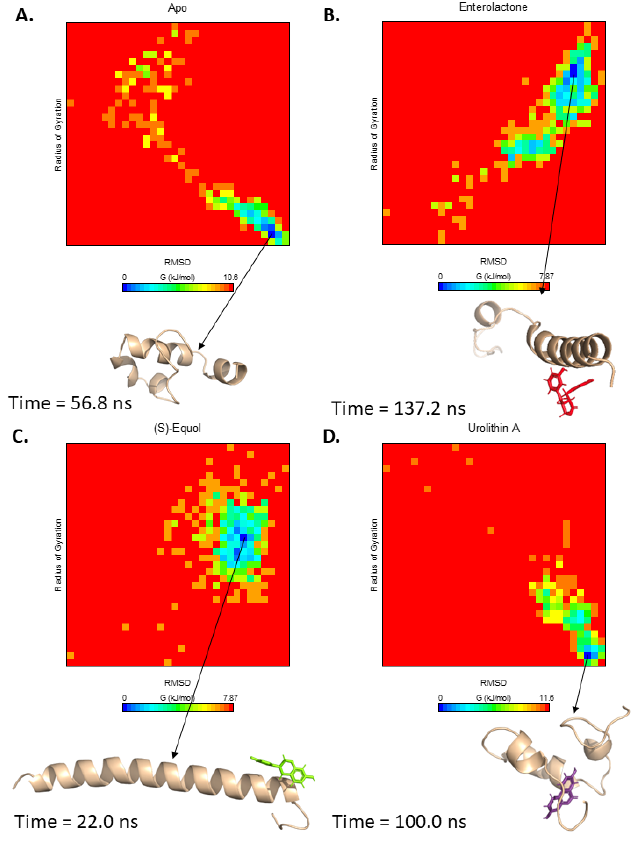
These results can be explained by the fact that urolithin A can form more stable interactions with Aβ42 residues in its meta-stable state than enterolactone or (S)-equol (Fig. 6). Specifically, in the meta-stable state, enterolactone can form both conventional and carbon-hydrogen bonds with Asp7, an amide-pi stacked interaction and a carbon-hydrogen bond with Gly9, 2 pi-pi stacked interactions with Tyr10 and His13, and a van der Waals interaction with His6. (S)-equol forms five van der Waals interactions with Asp7, Ser8, Glu11, Val12, and Gln15. Conversely, urolithin A, with many more interactions, can form eight van der Waals interactions (with Glu11, Lys16, Phe20, Met35, Val36, Gly37, Gly38, and Val 39), one pi-pi stacked interaction with Phe19, and two pi-alkyl interactions with Val40 and Ile41.
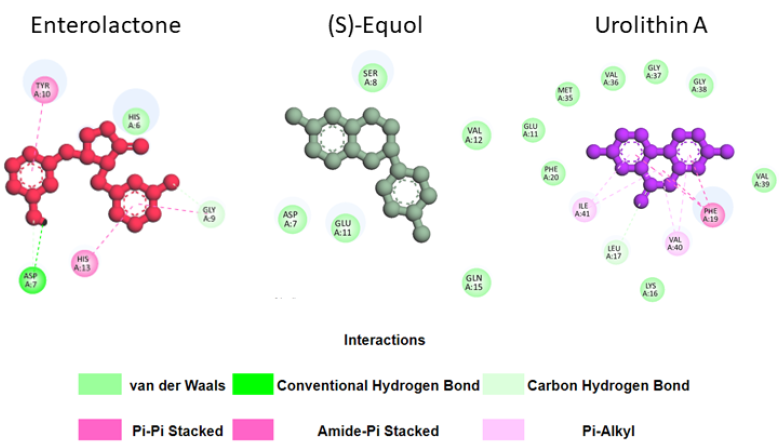
MM/PBSA binding energy analyses of Aβ42 complexes
Evaluating the binding affinities of the ligands by employing the MM/PBSA method offers enhanced validity to the simulated interaction (Fig. 7). Enterolactone exhibited the strongest binding affinity at -291.0 kJ/mol, while (S)-equol and urolithin A demonstrated weaker binding affinities of -60.0 and -83.6 kJ/mol, respectively. The solvation energies were generally comparable among the three complexes (-3569.6, -3313.60, and -3232.50 kJ/mol, for enterolactone, (S)-equol, and urolithin A complex, respectively). The ligand solvation energies for enterolactone, (S)-equol, and urolithin A were -96.2, -80.10, and -133.50 kJ/mol, respectively. The receptor solvation energies for enterolactone, (S)-equol, and urolithin A were -3246.5, -3240.96, and -3124.50 kJ/mol, respectively. Interestingly, enterolactone displayed the highest potential complex, ligand, and receptor energies. The potential complex energies for enterolactone, (S)-equol, and urolithin A were 545.2, 125.60, and -163.40 kJ/mol, respectevely. The potential ligand energies for enterolactone, (S)-equol, and urolithin A were 63.0, -113.80, and -112.10 kJ/mol. Finally, the potential receptor energies for enterolactone, (S)-equol, and urolithin A were 546.7, 307.0, and 57.5 kJ/mol.
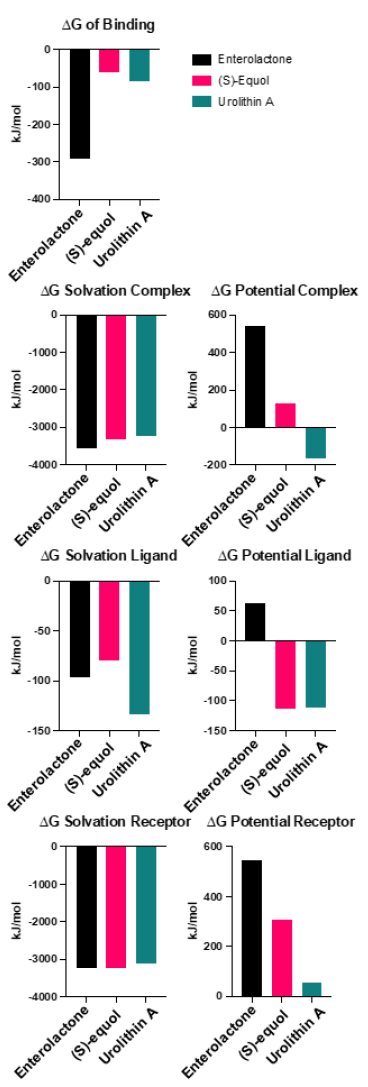
Discussion
The adoption of a U-shaped conformation (bringing its C-terminus and N-terminus into close proximity) is one of the key indicators of Aβ42’s predisposition to form plaques or neurofibrillary tangles, and this phenomenon has been substantiated in prior studies, both through computational simulations and biochemical experiments [32][41]. Notably, it has been demonstrated in silico that Aβ42 achieves this “ready-to-polymerize” state in less than 100 ns [32], which is similar to the current simulation results. Furthermore, the free energy landscape analysis for apo-Aβ42 corroborates this, indicating that the peptide attains this favorable state in under 60 ns, which validates the initial setups of the current study. In this context, enterolactone and (S)-equol prevented the conformational transition of Aβ42 throughout the simulation. In contrast, urolithin A did not show similar potential during molecular dynamics simulation.
The examination of protein secondary structure revealed a significant reduction in coil structures and an elevation in turn structures in enterolactone and (S)-equol complexes in comparison to the apo-form. This observation suggests that enterolactone and (S)-equol have the potential to induce structural perturbations in the Aβ42 peptide. These data are consistent to some extent with the findings of [32], which indicated an augmentation in the coil and turn conformation in the epigallocatechin-3-gallate-Aβ42 and genistein-Aβ42 complexes in silico when compared to the Aβ42 peptide. Furthermore, the interaction of Aβ42 with the metabolites led to reduced intra-connectivity, as evidenced by the decrease in strongly correlated residue pairs and the complete absence of strongly anti-correlated residue pairs. This observation indicates that the metabolites may inhibit Aβ42, resulting in reduced conformational fluctuations and flexibility. Interestingly, the free energy landscape analysis revealed that the Aβ42-urolithin A complex required a higher amount of energy to reach the most unstable state, indicating increased stability compared to the enterolactone and (S)-equol complexes. This observation is attributed to the higher number of stable interactions formed by urolithin A with Aβ42 residues in the meta-stable state.
The MM/PBSA binding affinity calculations indicated that enterolactone displayed the strongest binding affinity with Aβ42 (-291.0 kJ/mol) while urolithin A demonstrated the weakest binding affinity. Additionally, the high potential complex, ligand, and receptor energies signify the favorable interaction between enterolactone and Aβ42. Therefore, enterolactone showed promise in inhibiting Aβ42, while urolithin A may not offer the same protective effects.
The preceding observations pertain to the putative inhibitory effect of enterolactone against Aβ42. The results underscore the potential significance of diets rich in matairesinol in inhibiting the action of Aβ42, since enterolactone is a matairesinol-derrived metabolite generated through the activity of gut microbiota. However, it is important to note that the current research does not advocate for this approach as a sole clinical treatment or an alternative for allopathic medicine; rather, it is positioned as a preventive and complementary medicine strategy. Nevertheless, it is imperative to conduct confirmatory experimental studies in order to authenticate the existing findings. Additionally, further investigation is required to comprehend the precise mechanisms that underlie these connections and to devise efficacious complementary therapeutic approaches that rely on the manipulation of gut microbiota and their metabolites.
References
1 | Bomasang-Layno E, Bronsther R. Diagnosis and Treatment of Alzheimer’s Disease:: An Update. Del. J. Public Health. 2021;7(4):74–85. DOI |
2 | Murphy MP, LeVine III H. Alzheimer’s disease and the amyloid-β peptide. Journal of Alzheimer’s disease. 2010;19(1):311-23. DOI |
3 | Gaya P, Medina M, Sánchez-Jiménez A, Landete JM. Phytoestrogen metabolism by adult human gut microbiota. Molecules. 2016;21(8):1034. DOI |
4 | Gaya P, Sánchez-Jiménez A, Peirotén Á, Medina M, Landete JM. Incomplete metabolism of phytoestrogens by gut microbiota from children under the age of three. Int. J. Food Sci. Nutr. 2018;69(3):334–43. DOI |
5 | Liggins J, Bluck LJ, Runswick S, Atkinson C, Coward WA, Bingham SA. Daidzein and genistein content of fruits and nuts. J. Nutr. Biochem. 2000;11(6):326–31. DOI |
6 | Milder IEJ, Feskens EJM, Arts ICW, Bueno de Mesquita HB, Hollman PCH, Kromhout D. Intake of the plant lignans secoisolariciresinol, matairesinol, lariciresinol, and pinoresinol in Dutch men and women. J. Nutr. 2005;135(5):1202–7. DOI |
7 | Sharifi-Rad J, Quispe C, Castillo CM, Caroca R, Lazo-Vélez MA, Antonyak H, Polishchuk A, Lysiuk R, Oliinyk P, De Masi L, Bontempo P. Ellagic acid: A review on its natural sources, chemical stability, and therapeutic potential. Oxid. Med. Cell. Longev. 2022. DOI |
8 | Francini-Pesenti F, Spinella P, Calò LA. Potential role of phytochemicals in metabolic syndrome prevention and therapy. Diabetes Metab. Syndr. Obes. Targets Ther. 2019;12:1987–2002. DOI |
9 | Kim H, Caulfield LE, Garcia-Larsen V, Steffen LM, Coresh J, Rebholz CM. Plant-Based Diets Are Associated With a Lower Risk of Incident Cardiovascular Disease, Cardiovascular Disease Mortality, and All-Cause Mortality in a General Population of Middle-Aged Adults. J. Am. Heart. Assoc. 2019;8(16):e012865. DOI |
10 | Molina-Montes E, Salamanca-Fernández E, Garcia-Villanova B, Sánchez MJ. The Impact of Plant-Based Dietary Patterns on Cancer-Related Outcomes: A Rapid Review and Meta-Analysis. Nutrients. 2020;12(7):2010. DOI |
11 | Gu Y, Scarmeas N. Dietary patterns in Alzheimer’s disease and cognitive aging. Curr. Alzheimer Res. 2011;8(5):510–9. DOI |
12 | Omar SH, Scott CJ, Hamlin AS, Obied HK. The protective role of plant biophenols in mechanisms of Alzheimer’s disease. J. Nutr. Biochem. 2017;47:1–20. DOI |
13 | Román GC, Jackson RE, Gadhia R, Román AN, Reis J. Mediterranean diet: The role of long-chain ω-3 fatty acids in fish; polyphenols in fruits, vegetables, cereals, coffee, tea, cacao and wine; probiotics and vitamins in prevention of stroke, age-related cognitive decline, and Alzheimer disease. Rev. Neurol. 2019;175(10):724–41. DOI |
14 | Zhang YJ, Gan RY, Li S, Zhou Y, Li AN, Xu DP, Li HB. Antioxidant phytochemicals for the prevention and treatment of chronic diseases. Molecules. 2015;20(12):21138-56. DOI |
15 | Szymanska R, Pospisil P, Kruk J. Plant-Derived Antioxidants in Disease Prevention. Oxid Med Cell Longev. 2016;2016:1920208. DOI |
16 | Shin SA, Joo BJ, Lee JS, Ryu G, Han M, Kim WY, Park HH, Lee JH, Lee CS. Phytochemicals as Anti-Inflammatory Agents in Animal Models of Prevalent Inflammatory Diseases. Molecules. 2020;25(24):5932. DOI |
17 | Di Sotto A, Vitalone A, Di Giacomo S. Plant-Derived Nutraceuticals and Immune System Modulation: An Evidence-Based Overview. Vaccines. 2020;8(3):468. DOI |
18 | Porzoor A, Alford B, Hügel HM, Grando D, Caine J, Macreadie I. Anti-amyloidogenic properties of some phenolic compounds. Biomolecules. 2015;5(2):505–27. DOI |
19 | Velander P, Wu L, Henderson F, Zhang S, Bevan DR, Xu B. Natural product-based amyloid inhibitors. Biochem. Pharmacol. 2017;139:40–55. DOI |
20 | Kujawska M, Jodynis-Liebert J. Potential of the ellagic acid-derived gut microbiota metabolite – Urolithin A in gastrointestinal protection. World J. Gastroenterol. 2020;26(23):3170–81. DOI |
21 | Li Y, Wang F, Li J, Ivey KL, Wilkinson JE, Wang DD, Li R, Liu G, Eliassen HA, Chan AT, Clish CB. Dietary lignans, plasma enterolactone levels, and metabolic risk in men: exploring the role of the gut microbiome. BMC Microbiol. 2022;22(1):82. DOI |
22 | Mayo B, Vázquez L, Flórez AB. Equol: A Bacterial Metabolite from The Daidzein Isoflavone and Its Presumed Beneficial Health Effects. Nutrients. 2019;11(9):2231. DOI |
23 | Mezhibovsky E, Hoang SH, Szeto S, Roopchand DE. In silico analysis of dietary polyphenols and their gut microbial metabolites suggest inhibition of SARS-CoV-2 infection, replication, and host inflammatory mediators. J. Biomol. Struct. Dyn. 2023. DOI |
24 | Zeleniuch-Jacquotte A, Adlercreutz H, Shore RE, Koenig KL, Kato I, Arslan AA, Toniolo P. Circulating enterolactone and risk of breast cancer: a prospective study in New York. Br. J. Cancer. 2004;91(1):99–105. DOI |
25 | Mali AV, Padhye SB, Anant S, Hegde MV, Kadam SS. Anticancer and antimetastatic potential of enterolactone: Clinical, preclinical and mechanistic perspectives. Eur. J. Pharmacol. 2019;852:107–24. DOI |
26 | Utian WH, Jones M, Setchell KD. S-equol: A potential nonhormonal agent for menopause-related symptom relief. J Womens Health. 2015;24(3):200-8. DOI |
27 | García‐Villalba R, Giménez‐Bastida JA, Cortés‐Martín A, Ávila‐Gálvez MÁ, Tomás‐Barberán FA, Selma MV, Espín JC, González‐Sarrías A. Urolithins: a Comprehensive Update on their Metabolism, Bioactivity, and Associated Gut Microbiota. Mol. Nutr. Food. Res. 2022;66(21):e2101019. DOI |
28 | Djedjibegovic J, Marjanovic A, Panieri E, Saso L. Ellagic Acid-Derived Urolithins as Modulators of Oxidative Stress. Oxid. Med. Cell. Longev. 2020;2020:5194508. DOI |
29 | Gong QY, Cai L, Jing Y, Wang W, Yang DX, Chen SW, Tian HL. Urolithin A alleviates blood-brain barrier disruption and attenuates neuronal apoptosis following traumatic brain injury in mice. Neural. Regen. Res. 2022;17(9):2007–13. DOI |
30 | Reddy VP, Aryal P, Robinson S, Rafiu R, Obrenovich M, Perry G. Polyphenols in Alzheimer’s Disease and in the Gut-Brain Axis. Microorganisms. 2020;8(2):199. DOI |
31 | Johnson SL, Park HY, Vattem DA, Grammas P, Ma H, Seeram NP. Equol, a Blood-Brain Barrier Permeable Gut Microbial Metabolite of Dietary Isoflavone Daidzein, Exhibits Neuroprotective Effects against Neurotoxins Induced Toxicity in Human Neuroblastoma SH-SY5Y Cells and Caenorhabditis elegans. Plant Foods Hum. Nutr. Dordr. Neth. 2020;75(4):512–7. DOI |
32 | Fang M, Zhang Q, Wang X, Su K, Guan P, Hu X. Inhibition Mechanisms of (-)-Epigallocatechin-3-gallate and Genistein on Amyloid-beta 42 Peptide of Alzheimer’s Disease via Molecular Simulations. ACS Omega. 2022;7(23):19665–75. DOI |
33 | El Shatshat A, Pham AT, Rao PPN. Interactions of polyunsaturated fatty acids with amyloid peptides Aβ40 and Aβ42. Arch. Biochem. Biophys. 2019;663:34–43. DOI |
34 | Liu S, Gao J, Zhu M, Liu K, Zhang HL. Gut Microbiota and Dysbiosis in Alzheimer’s Disease: Implications for Pathogenesis and Treatment. Mol. Neurobiol. 2020;57(12):5026–43. DOI |
35 | Cerovic M, Forloni G, Balducci C. Neuroinflammation and the Gut Microbiota: Possible Alternative Therapeutic Targets to Counteract Alzheimer’s Disease? Front. Aging Neurosci. 2019;11:284. DOI |
36 | Chen C, Ahn EH, Kang SS, Liu X, Alam A, Ye K. Gut dysbiosis contributes to amyloid pathology, associated with C/EBPβ/AEP signaling activation in Alzheimer’s disease mouse model. Sci. Adv. 2020;6(31):eaba0466. DOI |
37 | Land H, Humble MS. YASARA: a tool to obtain structural guidance in biocatalytic investigations. In: Protein Engineering. Springer. 2018:43–67. DOI |
38 | Hoang SH. Fibroblast growth factor 5 (FGF5) and its missense mutant FGF5-H174 underlying trichomegaly: a molecular dynamics simulation investigation. J. Biomol. Struct. Dyn. 2023. DOI |
39 | Hoang SH, Dao H, Lam EM. A network pharmacology approach to elucidate the anti-inflammatory effects of ellagic acid. J. Biomol. Struct. Dyn. 2023. DOI |
40 | Duan J, Li Y, Gao H, Yang D, He X, Fang Y, Zhou G. Phenolic compound ellagic acid inhibits mitochondrial respiration and tumor growth in lung cancer. Food. Funct. 2020;11(7):6332–9. DOI |
41 | Colvin MT, Silvers R, Ni QZ, Can TV, Sergeyev I, Rosay M, Donovan KJ, Michael B, Wall J, Linse S, Griffin RG. Atomic Resolution Structure of Monomorphic Aβ42 Amyloid Fibrils. J. Am. Chem. Soc. 2016;138(30):9663–74. DOI |
Cite this article:
Hoang, S., Lam, E., Dao, H. Computational assessment of gut microbiota metabolite enterolactone as a promising Aβ42 inhibitor in Alzheimer’s disease. DYSONA – Life Science, 2024;5(1): 9-20. doi: 10.30493/dls.2023.419136