Michael Alabboud 1,2*
1, Department of Horticulture, Al-Baath University, Homs, Syria
2, Department of Horticultural Sciences, UTCAN, University of Tehran, Iran
E-mail:
alabboud@ut.ac.ir
Received: 30/01/2022
Acceptance: 10/02/2022
Available Online: 11/02/2022
Published: 01/04/2022
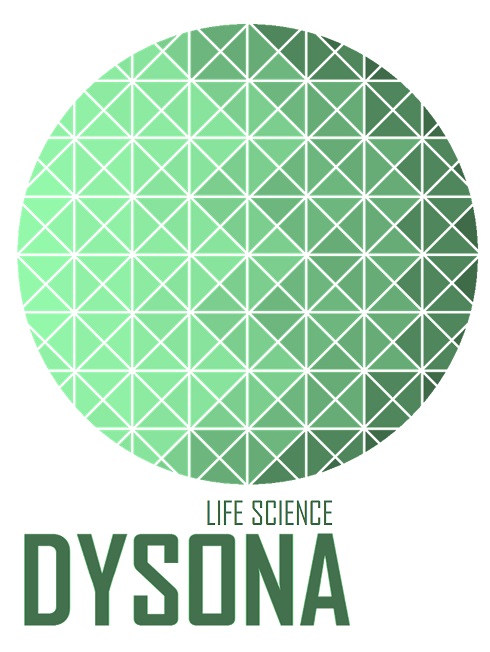
Manuscript link
http://dx.doi.org/10.30493/DLS.2022.329268
Abstract
The role that deep learning plays in modern life is undeniably essential. It is also certain that deep learning, with its various approaches, is contributing significantly to plant science. Whether by explaining the acquired data or converting and refining these data to a more profound level, deep learning techniques are pushing the frontiers of plant research further than ever before. This study is an attempt to shed light on recent advances and applications of deep learning in plant science. These applications were systematically reviewed at omics, micro/macroscopic, and population levels. Future aspects were also discussed to some extent.
Keywords: Convolutional neural networks, Deep learning applications, Plant omics
Introduction
Artificial intelligence (AI) is defined as the act of thinking and learning when performed by a computer program or machine. Due to its capability of simulating human intelligence and thus performing complicated human tasks, AI is steadily replacing human forces in various sectors. In science, AI is creating new frontiers in environmental [1], medical [2], pharmaceutical [3], biological [4], agricultural [5], and engineering [6] sciences.
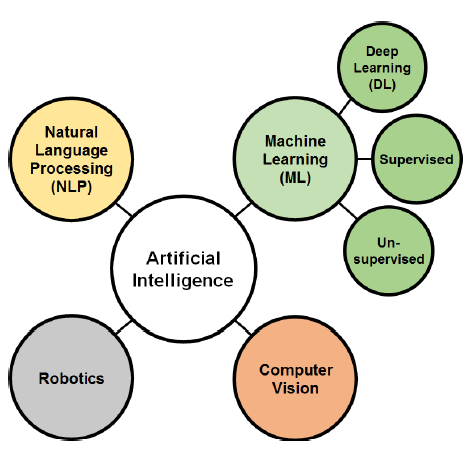
Various methodologies are used in AI research, and classifying these methods is tricky since many of them are interconnected. However, the classification agreed upon by many scholars subdivides AI techniques into four divisions: Machine learning (ML), Natural language processing (NLP), Computer vision, and Robotics (Fig. 1). ML is a major branch of AI that gained increasing importance in the big data era [7]. Deep learning (DL) is a subset field of ML (Fig. 1). The recent rapid growth of DL is generated from the need for a real-time image processing technique, which is the main application of DL through deep and convolutional neural networks (CNN).
Deep learning models are developed to tackle real-life problems in various scientific fields. The aim of this study was to review recent literature of DL applications in plant science. Furthermore, the major difficulties and future aspects of DL in plant science will be discussed.
Deep learning definition
Deep learning is a comprehensive tool of ML that is constituted upon the principles of artificial neural networks (ANN) with great capability to discover complex features in multilayered data. In fact, the main advantage of DL technique compared to conventional ANNs is its efficiency in automatically extracting the significant features of the analyzed data. This automation allows the researcher to focus on model architecture and results reasoning rather than spending long hours on manual feature extraction [8]. This advantage rendered DL as an ideal tool to tackle many scientific problems in health care [9], bioinformatics [10], finance [11], and agriculture [12].
Deep learning in plant science
DL potentials in plant science research are undeniable. The reason behind this certainty is that plant science, similar to all modern sciences, dramatically benefits from visual data and data visualization [13]. Technically, any form of visual input can be used for this technique, whether it is any form of spectral imaging or visualization of data to be interpreted as an image such as metabolic profiles and nucleic acid sequence. However, different DL techniques are suitable for the different data inputs. For instance, CNN models are ideal for high dimensional data such as spectral images inputs, while recurrent neural network (RNN) and long-/short-term memory (LSTM) are preferable for sequential data such as DNA and RNA sequences.
Many approaches can be used to tackle the subject of deep learning applications in plant science. For instance, DL applications can be classified technically based on the DL technique or input data format. However, the current review addresses the subject by classifying DL applications into three distinctive levels: Omics level, Micro/Macroscopic level, and population level.
Omics level
Omics are a collective field of biological studies that end with the suffix -omics, such as genomics, transcriptomics, proteomics, and metabolomics. These studies address the quantification, structure, and functionality of biological molecules. Therefore, omics provide valuable knowledge of plant organizational functionality [14]. Due to the large data usually provided by omics studies methodologies, DL became a necessary inseparable tool of omics data processing and reasoning.
Since omics data is usually sequential, RNNs and LSTM are widely used to process these data. The primary purpose of DL in omics studies is to locate and highlight unique features of interest in the studied data, such as detecting single nuclear polymorphisms (SNPs) (Fig. 2 A) and enhancers’ sequences (Fig. 2 B) [15] or to translate the obtained data (input) into other forms of information (output). For this purpose, molecular data is collectively or individually used to produce a general conceptualization of the plant morphology and phenotypic characteristics, which might have limitless breeding applications [16] (Fig. 2 C).
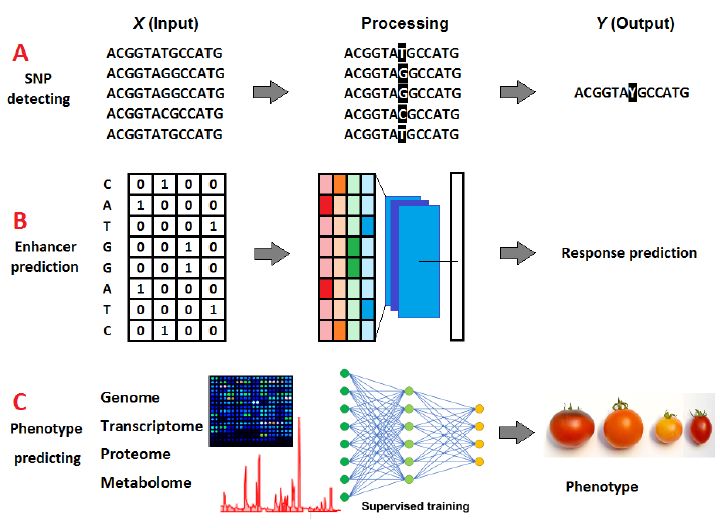
DL techniques are pushing omics research forward in many aspects (Table 1); however, it is stated that other techniques, such as non-additive Gaussian kernel or simple arc-cosine kernel, might produce more reliable predictions based on omics data compared to DL [17]. This observation might be attributed to the overall complicated DL fine-tuning. Therefore, more research should be conducted in the field of DL model’s optimization for omics-based predictions.
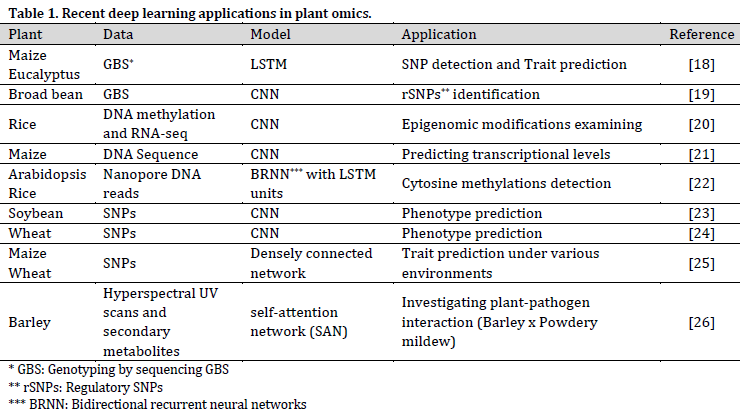
Micro/Macroscopic level
The main concern of microscopic studies is to investigate the plant on a cellular level, such as cellular organelles, full cell, and tissue research. On the other hand, macroscopic studies, in this context, address the characterizations and interactions of a whole or part of a plant. The data used for this type of study are predominantly images of any range of the electromagnetic spectrum.
Various data acquisition systems are being used in Micro/Macroscopic DL, such as visible light sensors [27], infrared (IR) and near-infrared (NIR) [28], ultraviolet [29], hyperspectral [30], and even X-rays [31]. Furthermore, other supportive techniques are being used to increase the depth of the acquired imagery such as cell staining in microscopy [32] and fluorescence imaging systems [33]. There are also some attempts to employ plant’s electrical signals measurements in DL research [34].
The applications of DL in this level are countless such as taxonomy and classification, disease/stress recognition and early warning systems, and physiological events tracking. (Table 2) covers some of the recent studies in the field.
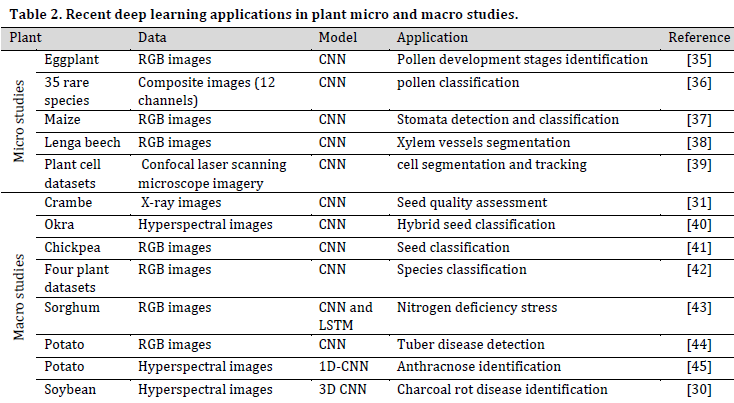
Population level
Population plant science refers to the study of plant communities, whether in natural habitats (e.g., forests, grasslands, and deserts) or in agricultural land. Population studies tackle various issues such as plant cover classification and surveys, plant communities’ biological functions, the interactions between individuals or species, and how plant populations are being influenced and influence the surrounding environment (Table 3).
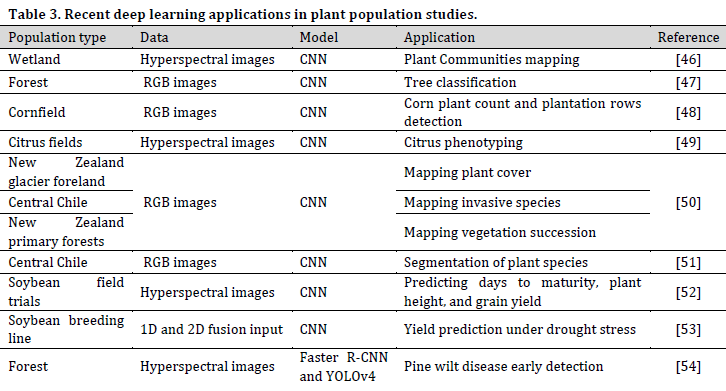
Spatial imagery is the predominant study material in DL population studies. The exponential increase in the availability of remote sensing (RS) datasets increased DL dependant plant population studies. Furthermore, the recent developments in unmanned aerial vehicles (UAV) manufacturing resulted in the production of lighter, more powerful, and affordable drones capable of carrying all sorts of image acquiring systems. These developments created a precious opportunity for research teams to create their RS datasets.
Current and future aspects of deep learning in plant science
Although DL can provide viable solutions to many complicated issues in plant research, the application of DL in plant science studies is still faced with many obstacles. One of the hurdles facing DL in plant science is dataset size and availability. Due to the relatively high costs of omics research, and the labors related to all three discussed levels of plant research, constructing largely enough datasets is a tiresome task. In fact, DL models depend greatly on the size and balance of the training and validating datasets to illustrate adequate generalization since small datasets usually result in overfitted models [55]. To overcome this obstacle, image augmentation techniques (Fig. 3) are used to expand the dataset size. Various researchers employed data augmentation to increase the accuracy of the developed DL models to some extent. However, increasing dataset size by adding new samples is still required to obtain more reliable results, especially in omics studies, where augmentation techniques are inapplicable.

On the other hand, large datasets require long hours of human supervised labeling since the training process requires adequately labeled data. However, the current rise of self-supervised learning (SSL) and semi-supervised learning might provide a viable solution to this problem since these techniques require unlabeled or partially labeled datasets to learn [56].
Among all three discussed levels of DL research in plant science, omics are still poorly represented. This poor representation is mainly due to the high costs of datasets generating and the unsuitability of omics data in its raw forms to be used in DL training which requires long hours of processing. Therefore, developing new methods for omics data preparation and pre-processing is necessary. Furthermore, employing layer visualizing methods such as saliency map [23] and feature map [27] might be of great importance. These maps provide valuable information regarding the features CNNs use to classify and predict. Therefore, these visualizations can assist omics research in selecting the best plant characteristics.
As for micro/macroscopic levels, it is expected that DL techniques will transform from a complementary assistant tool to play more vital roles. Novel DL models are being developed daily to provide a deeper understanding of physiological processes and biological interactions between plants and their abiotic and biotic surroundings [43-45]. Additionally, DL has excellent potential to provide rapid and accurate judgments in agricultural production lines [27].
Population level DL studies are expected to play significant roles in the real-time tracking of invasive species and plant population dynamics in natural habitats [46][47][50][51]. Furthermore, the new models will introduce a new age of cost-effective and accurate DL-assisted agricultural extension [52][53], which will significantly positively impact food production chains in the near future.
References
1 | Gupta PK, Saxena A, Dattaprakash B, Sheriff RS, Chaudhari SH, Ullanat V, Chayapathy V. Applications of Artificial Intelligence in Environmental Science. Artif. Intell. 2021:225-40. DOI |
2 | Mainali G. Artificial Intelligence in Medical Science: Perspective from a Medical Student. JNMA J Nepal Med Assoc. 2020;58(229):709. DOI |
3 | Mak KK, Pichika MR. Artificial intelligence in drug development: present status and future prospects. Drug Discov. Today. 2019;24(3):773-80. DOI |
4 | Han H, Liu W. The coming era of artificial intelligence in biological data science. BMC Bioinform. 2019;20(22):1-2. DOI |
5 | Misra NN, Dixit Y, Al-Mallahi A, Bhullar MS, Upadhyay R, Martynenko A. IoT, big data and artificial intelligence in agriculture and food industry. IEEE Internet of Things Journal. 2020. DOI |
6 | Nti IK, Adekoya AF, Weyori BA, Nyarko-Boateng O. Applications of artificial intelligence in engineering and manufacturing: A systematic review. J. Intell. Manuf. 2021:1-21. DOI |
7 | Liakos KG, Busato P, Moshou D, Pearson S, Bochtis D. Machine learning in agriculture: A review. Sensors. 2018;18(8):2674. DOI |
8 | Ren P, Xiao Y, Chang X, Huang PY, Li Z, Gupta BB, Chen X, Wang X. A survey of deep active learning. ACM Comput. Surv. 2021;54(9):1-40. DOI |
9 | Esteva A, Robicquet A, Ramsundar B, Kuleshov V, DePristo M, Chou K, Cui C, Corrado G, Thrun S, Dean J. A guide to deep learning in healthcare. Nat. Med. 2019;25(1):24-9. DOI |
10 | Cao Y, Geddes TA, Yang JY, Yang P. Ensemble deep learning in bioinformatics. Nat. Mach. Intell. 2020;2(9):500-8. DOI |
11 | Heaton JB, Polson NG, Witte JH. Deep learning for finance: deep portfolios. Appl. Stoch. Models Bus. Ind. 2017;33(1):3-12. DOI |
12 | Kamilaris A, Prenafeta-Boldú FX. Deep learning in agriculture: A survey. Comput Electron Agric. 2018;147:70-90. DOI |
13 | Alnjar HR. Data visualization metrics between theoretic view and real implementations: A review. DYSONA Appl. Sci. 2020;1(2):43-50. DOI |
14 | Hakeem KR, Tombuloğlu H, Tombuloğlu G, editors. Plant omics: trends and applications. Basel, Switzerland Springer. 2016. DOI |
15 | Min X, Chen N, Chen T, Jiang R. DeepEnhancer: Predicting enhancers by convolutional neural networks. In2016 IEEE International Conference on Bioinformatics and Biomedicine (BIBM). 2016:637-44. DOI |
16 | Guo W, Xu Y, Feng X. DeepMetabolism: a deep learning system to predict phenotype from genome sequencing. arXiv preprint arXiv:1705.03094. 2017. |
17 | Crossa J, Martini JW, Gianola D, Pérez-Rodríguez P, Jarquin D, Juliana P, Montesinos-López O, Cuevas J. Deep kernel and deep learning for genome-based prediction of single traits in multienvironment breeding trials. Front. Genet. 2019;10:1168. DOI |
18 | Maldonado C, Mora F, Contreras-Soto R, Ahmar S, Chen JT, do Amaral Júnior AT, Scapim CA. Genome-wide prediction of complex traits in two outcrossing plant species through Deep Learning and Bayesian Regularized Neural Network. Front. Plant Sci. 2020;11:1734. DOI |
19 | Heinrich F, Wutke M, Das PP, Kamp M, Gültas M, Link W, Schmitt AO. Identification of regulatory SNPs associated with vicine and convicine content of Vicia faba based on genotyping by sequencing data using deep learning. Genes. 2020;11(6):614. DOI |
20 | Wang Y, Zhang P, Guo W, Liu H, Li X, Zhang Q, Du Z, Hu G, Han X, Pu L, Tian J. A deep learning approach to automate whole‐genome prediction of diverse epigenomic modifications in plants. New Phytol. 2021;232(2):880-97. DOI |
21 | Washburn JD, Mejia-Guerra MK, Ramstein G, Kremling KA, Valluru R, Buckler ES, Wang H. Evolutionarily informed deep learning methods for predicting relative transcript abundance from DNA sequence. Proc. Natl. Acad. Sci. U.S.A. 2019;116(12):5542-9. DOI |
22 | Ni P, Huang N, Nie F, Zhang J, Zhang Z, Wu B, Bai L, Liu W, Xiao CL, Luo F, Wang J. Genome-wide detection of cytosine methylations in plant from Nanopore data using deep learning. Nat. Commun. 2021;12(1). DOI |
23 | Liu Y, Wang D, He F, Wang J, Joshi T, Xu D. Phenotype prediction and genome-wide association study using deep convolutional neural network of soybean. Front. Genet. 2019;10:1091. DOI |
24 | Sandhu KS, Lozada DN, Zhang Z, Pumphrey MO, Carter AH. Deep learning for predicting complex traits in spring wheat breeding program. Front. Plant Sci. 2021;11:2084. DOI |
25 | Montesinos-López OA, Montesinos-López A, Crossa J, Gianola D, Hernández-Suárez CM, Martín-Vallejo J. Multi-trait, multi-environment deep learning modeling for genomic-enabled prediction of plant traits. G3-GENES GENOM GENET. 2018;8(12):3829-40. DOI |
26 | Brugger A, Schramowski P, Paulus S, Steiner U, Kersting K, Mahlein AK. Spectral signatures in the UV range can be combined with secondary plant metabolites by deep learning to characterize barley–powdery mildew interaction. Plant Pathol. 2021;70(7):1572-82. DOI |
27 | Alabboud M, Kalantari S, Soltani F. Novel models to predict stored melon fruit marketability using convolutional neural networks. J Ambient Intell Humaniz Comput. 2022 (Accepted manuscript) |
28 | Gao Z, Luo Z, Zhang W, Lv Z, Xu Y. Deep learning application in plant stress imaging: a review. AgriEngineering. 2020;2(3):430-46. DOI |
29 | Sabanci K, Aslan MF, Durdu A. Bread and durum wheat classification using wavelet based image fusion. J. Sci. Food Agric. 2020;100(15):5577-85. DOI |
30 | Nagasubramanian K, Jones S, Singh AK, Sarkar S, Singh A, Ganapathysubramanian B. Plant disease identification using explainable 3D deep learning on hyperspectral images. Plant methods. 2019;15(1):1-0. DOI |
31 | de Medeiros AD, Bernardes RC, da Silva LJ, de Freitas BA, dos Santos Dias DC, da Silva CB. Deep learning-based approach using X-ray images for classifying Crambe abyssinica seed quality. Ind. Crops Prod. 2021;164:113378. DOI |
32 | Biswas S, Barma S. A large-scale optical microscopy image dataset of potato tuber for deep learning based plant cell assessment. Sci. Data. 2020;7(1). DOI |
33 | Sun D, Zhu Y, Xu H, He Y, Cen H. Time-series chlorophyll fluorescence imaging reveals dynamic photosynthetic fingerprints of sos mutants to drought stress. Sensors. 2019;19(12):2649. DOI |
34 | Tran D, Dutoit F, Najdenovska E, Wallbridge N, Plummer C, Mazza M, Raileanu LE, Camps C. Electrophysiological assessment of plant status outside a Faraday cage using supervised machine learning. Sci. Rep. 2019;9(1):1-9. DOI |
35 | García-Fortea E, García-Pérez A, Gimeno-Páez E, Sánchez-Gimeno A, Vilanova S, Prohens J, Pastor-Calle D. a deep learning-based system (microscan) for the identification of pollen development stages and its application to obtaining doubled haploid lines in Eggplant. Biology. 2020;9(9):272. DOI |
36 | Dunker S, Motivans E, Rakosy D, Boho D, Mäder P, Hornick T, Knight TM. Pollen analysis using multispectral imaging flow cytometry and deep learning. New Phytol. 2021;229(1):593-606. DOI |
37 | Aono AH, Nagai JS, Dickel GD, Marinho RC, de Oliveira PE, Papa JP, Faria FA. A stomata classification and detection system in microscope images of maize cultivars. PLoS One. 2021;16(10):e0258679. DOI |
38 | Garcia-Pedrero A, García-Cervigón A, Caetano C, Calderón-Ramírez S, Olano JM, Gonzalo-Martín C, Lillo-Saavedra M, García-Hidalgo M. Xylem vessels segmentation through a deep learning approach: a first look. In2018 IEEE international work conference on bioinspired intelligence (IWOBI). 2018. DOI |
39 | Jiang W, Wu L, Liu S, Liu M. CNN-based two-stage cell segmentation improves plant cell tracking. Pattern Recognit. Lett. 2019;128:311-7. DOI |
40 | Nie P, Zhang J, Feng X, Yu C, He Y. Classification of hybrid seeds using near-infrared hyperspectral imaging technology combined with deep learning. Sens. Actuators B Chem. 2019;296:126630. DOI |
41 | Taheri-Garavand A, Nasiri A, Fanourakis D, Fatahi S, Omid M, Nikoloudakis N. Automated in situ seed variety identification via deep learning: a case study in chickpea. Plants. 2021;10(7):1406. DOI |
42 | Kaya A, Keceli AS, Catal C, Yalic HY, Temucin H, Tekinerdogan B. Analysis of transfer learning for deep neural network based plant classification models. Comput Electron Agric. 2019;158:20-9. DOI |
43 | Rojanarungruengporn K, Pumrin S. Early Stress Detection in Plant Phenotyping using CNN and LSTM Architecture. In2021 9th International Electrical Engineering Congress (iEECON). 2021:389-92. DOI |
44 | Oppenheim D, Shani G, Erlich O, Tsror L. Using deep learning for image-based potato tuber disease detection. Phytopathology. 2019;109(6):1083-7. DOI |
45 | Liu F, Xiao Z. Disease Spots Identification of Potato Leaves in Hyperspectral Based on Locally Adaptive 1D-CNN. In2020 IEEE International Conference on Artificial Intelligence and Computer Applications (ICAICA) 2020:355-358. DOI |
46 | Du B, Mao D, Wang Z, Qiu Z, Yan H, Feng K, Zhang Z. Mapping Wetland Plant Communities Using Unmanned Aerial Vehicle Hyperspectral Imagery by Comparing Object/Pixel-Based Classifications Combining Multiple Machine-Learning Algorithms. IEEE Journal of Selected Topics in Applied Earth Observations and Remote Sensing. 2021;14:8249-58. DOI |
47 | Egli S, Höpke M. CNN-Based Tree Species Classification Using High Resolution RGB Image Data from Automated UAV Observations. Remote Sens. 2020;12(23):3892. DOI |
48 | Osco LP, de Arruda MD, Gonçalves DN, Dias A, Batistoti J, de Souza M, Gomes FD, Ramos AP, de Castro Jorge LA, Liesenberg V, Li J. A CNN approach to simultaneously count plants and detect plantation-rows from UAV imagery. ISPRS J. Photogramm. Remote Sens. 2021;174:1-17. DOI |
49 | Ampatzidis Y, Partel V. UAV-based high throughput phenotyping in citrus utilizing multispectral imaging and artificial intelligence. Remote Sens. 2019;11(4):410. DOI |
50 | Kattenborn T, Eichel J, Wiser S, Burrows L, Fassnacht FE, Schmidtlein S. Convolutional Neural Networks accurately predict cover fractions of plant species and communities in Unmanned Aerial Vehicle imagery. Remote. Sens. Ecol. Conserv. 2020;6(4):472-86. DOI |
51 | Kattenborn T, Eichel J, Fassnacht FE. Convolutional Neural Networks enable efficient, accurate and fine-grained segmentation of plant species and communities from high-resolution UAV imagery. Sci. Rep. 2019;9(1). DOI |
52 | Teodoro PE, Teodoro LP, Baio FH, da Silva Junior CA, dos Santos RG, Ramos AP, Pinheiro MM, Osco LP, Gonçalves WN, Carneiro AM, Junior JM. Predicting Days to Maturity, Plant Height, and Grain Yield in Soybean: A Machine and Deep Learning Approach Using Multispectral Data. Remote Sens. 2021;13(22):4632. DOI |
53 | Zhou J, Zhou J, Ye H, Ali ML, Chen P, Nguyen HT. Yield estimation of soybean breeding lines under drought stress using unmanned aerial vehicle-based imagery and convolutional neural network. Biosyst. Eng. 2021;204:90-103. DOI |
54 | Yu R, Luo Y, Zhou Q, Zhang X, Wu D, Ren L. Early detection of pine wilt disease using deep learning algorithms and UAV-based multispectral imagery. For. Ecol. Manag. 2021;497:119493. DOI |
55 | Salman S, Liu X. Overfitting mechanism and avoidance in deep neural networks. arXiv preprint arXiv:1901.06566. 2019. |
56 | Zhai X, Oliver A, Kolesnikov A, Beyer L. S4l: Self-supervised semi-supervised learning. InProceedings of the IEEE/CVF International Conference on Computer Vision 2019:1476-85. |
Cite this article:
Alabboud, M. Deep learning in plant science: A mini-review. DYSONA – Life Science, 2022;3(1): 7-15. doi: 10.30493/dls.2022.329268